The Potential of Data Labeling in Machine Learning: A Deep Dive
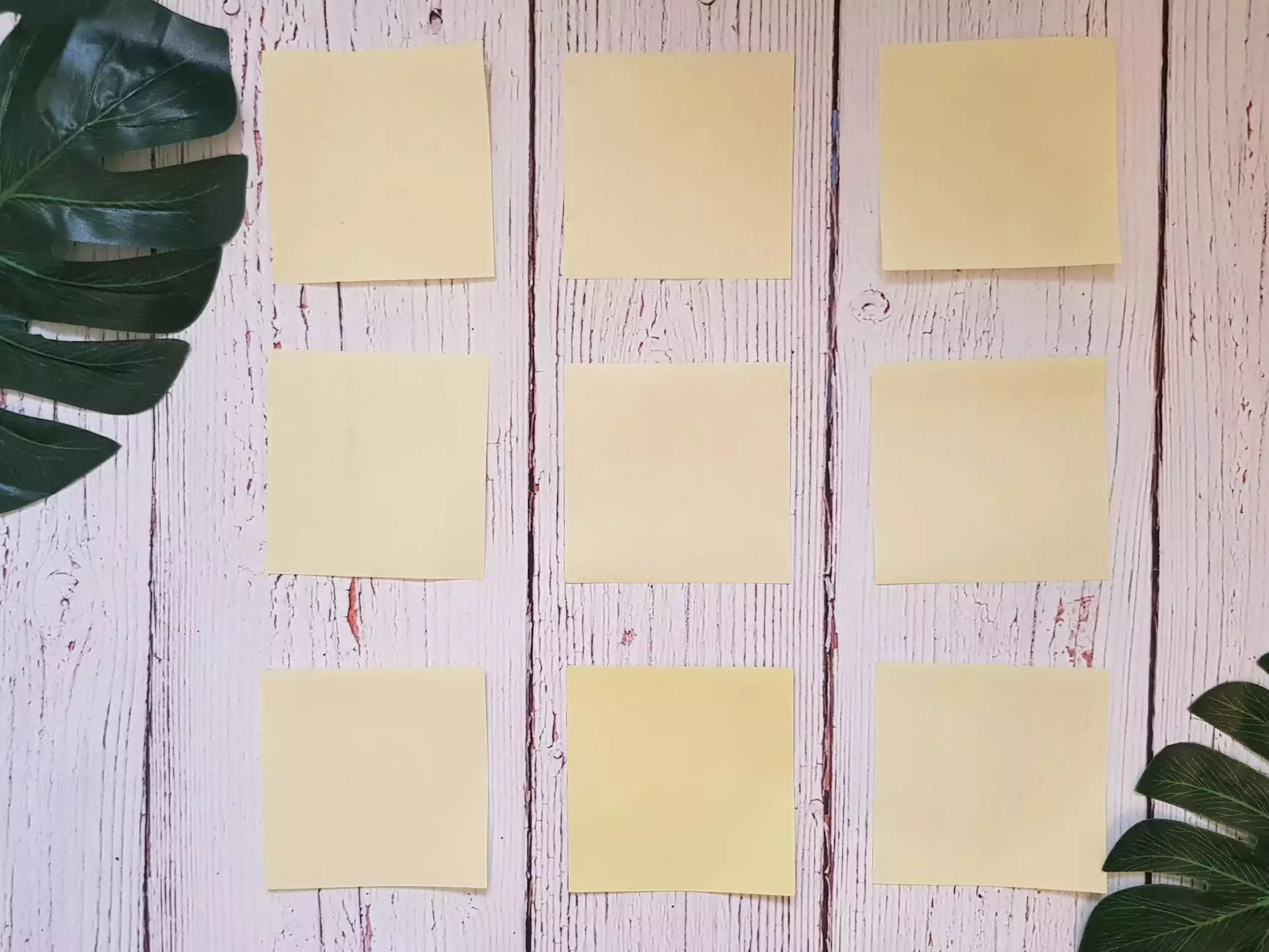
Understanding Data Labeling in Machine Learning
In the era of artificial intelligence and machine learning, the importance of data labeling cannot be overstated. Data labeling is the process of tagging or annotating data, which helps machine learning algorithms to understand and learn from that data. This foundational step is crucial to the success of any AI project, as it directly impacts the quality and reliability of the model being developed.
Data can take many forms including text, images, audio, and video, and labeling each type correctly is essential to creating a robust machine learning model. The practice of labeling and annotating data is key in various applications such as image recognition, natural language processing, and autonomous vehicles. In this article, we will explore the intricacies of data labeling in machine learning, the tools available through platforms like Keylabs.ai, and why proper annotation is critical for achieving successful outcomes.
Why Data Labeling Matters
The success of machine learning models is heavily dependent on the quality of data they are trained on. High-quality labeled data enhances a model's ability to make accurate predictions and classifications. Let's break down why data labeling matters:
- Improves Model Accuracy: Labeled data allows machine learning models to learn patterns and make correct predictions. Without accurate labels, models may produce unreliable outputs.
- Facilitates Supervised Learning: Most machine learning applications utilize supervised learning, where the model learns from previously labeled data. This requires extensive amounts of accurately annotated data.
- Enables Complex Applications: Many advanced applications, from medical diagnostics to facial recognition, rely on well-labeled data to deliver insights and results.
The Data Annotation Process
Data annotation is more than just tagging; it is a meticulous process that involves several steps:
- Data Collection: The first step is gathering raw data from various sources, which can include images, videos, or textual data.
- Data Preparation: Once collected, the data must be cleaned and preprocessed to ensure consistency and relevance.
- Labeling: This is the core of the annotation process where data is tagged using specific categories or labels. Each label corresponds to a feature or intention within the dataset.
- Quality Assurance: After labeling, a quality assurance check is necessary to verify the accuracy and consistency of the labels applied.
- Integration: The final step is integrating the labeled data into a machine learning framework for training and evaluation.
Keylabs.ai: Leading Data Annotation Platform
At Keylabs.ai, we offer a comprehensive data annotation platform that supports a wide array of data types. Our tools are designed to streamline the annotation process, making it efficient and effective for machine learning applications.
Here are some features that set Keylabs.ai apart:
- User-Friendly Interface: Our platform is designed to be intuitive, allowing users to label data quickly without sacrificing accuracy.
- Automated Annotation Tools: We leverage AI-driven tools that can assist in the annotation process, significantly reducing the time spent on repetitive tasks.
- Collaboration Features: Team members can work together seamlessly, sharing insights and results in real time.
- Customizable Workflows: Tailored workflows ensure that each project can be managed according to specific requirements and timelines.
- Robust Quality Checks: Our platform includes automated quality checks as well as options for manual verification to guarantee the highest label accuracy.
Best Practices for Data Labeling
To ensure that your data labeling is effective, here are some best practices to follow:
- Define Your Objectives: Clearly outline what you want to achieve with your data labeling project. This direction helps in annotating the data effectively.
- Establish Clear Guidelines: Create a comprehensive guide or manual that defines the labeling process and the meaning of each label.
- Train Your Labelers: Ensure that those involved in the labeling process are well-trained to understand both the subject matter and the labeling standards.
- Regularly Review and Update: Continuous improvement is crucial. Regular reviews and updates to your labeling guidelines can prevent work from becoming outdated.
- Use Technology Wisely: Leverage advanced tools and software for labeling, which can help speed up the process while maintaining accuracy.
The Future of Data Labeling in Machine Learning
As machine learning technologies evolve, so too will the methods and tools for data labeling. The convergence of artificial intelligence with data annotation is poised to revolutionize how we approach data labeling. We can expect further advancements in:
- Automated Annotation: Increased use of machine learning algorithms to automate labeling tasks, saving time and reducing human error.
- Real-Time Collaboration: Enhancements in platforms that allow for easier real-time collaboration and sharing among data scientists and labelers.
- Augmented Reality and Virtual Reality Data Annotation: As AR and VR continue to grow, new types of data require innovative annotation techniques.
- Ethical Considerations: The importance of ensuring that data is labeled without bias will become increasingly important as AI systems become more integral to business and society.
Conclusion
In summary, data labeling is an indispensable facet of machine learning. The quality of the labeled data directly correlates to the predictive performance of machine learning models. By utilizing platforms like Keylabs.ai, businesses can enhance their machine learning projects with high-quality data annotations. As we move forward, the integration of advanced technologies in the annotation process will not only optimize the labeling tasks but also lead to more intelligent and capable AI systems.
data labeling machine learning