The Future of IT: Harnessing Anti Malware Machine Learning
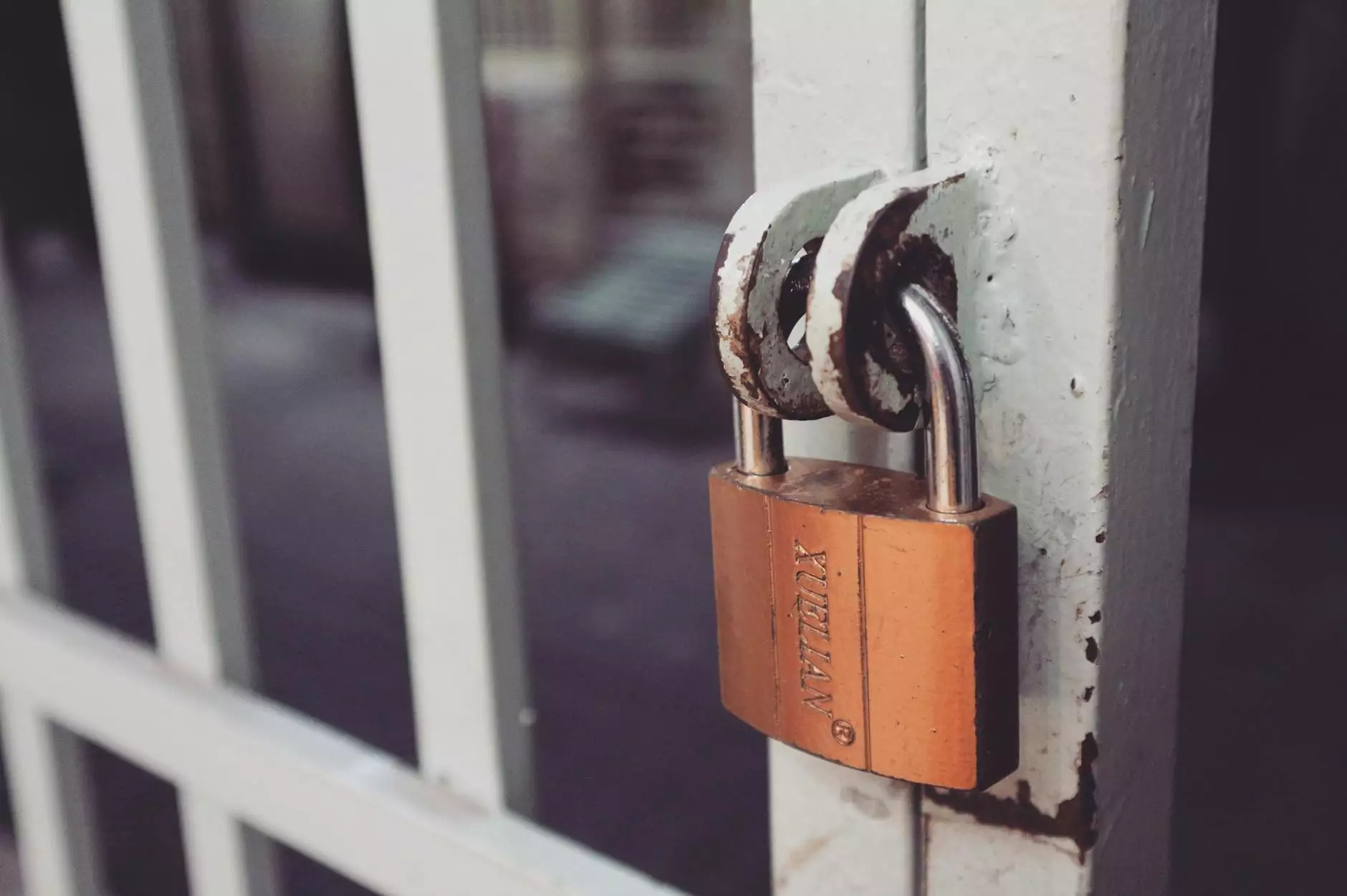
In today’s rapidly evolving digital landscape, the significance of robust cybersecurity measures cannot be overstated. The rise of cyber threats has led to a surge in demand for innovative solutions. One of the most promising advancements in this field is anti malware machine learning. This technology not only enhances security systems but also revolutionizes IT services and computer repair. In this article, we will delve into the intricacies of anti malware machine learning, its benefits, and practical applications for businesses looking to safeguard their digital assets.
Understanding Anti Malware Machine Learning
At its core, anti malware machine learning refers to the application of machine learning techniques to identify, analyze, and mitigate malware threats. Unlike traditional signature-based detection methods, which rely on a database of known malware signatures, machine learning enables systems to recognize patterns and anomalies indicative of malware behavior.
Key Components of Anti Malware Machine Learning
- Data Collection: Gathering data from various sources, including files, networks, and user behavior to train machine learning models.
- Feature Extraction: Identifying relevant features within the collected data that can indicate the presence of malware.
- Model Training: Using algorithms to train models that can detect patterns associated with both known and unknown malware.
- Real-Time Detection: Implementing trained models into security systems to provide real-time threat detection and response.
Why Machine Learning is Game-Changing for IT Security
The integration of machine learning in anti malware solutions is a game changer for several reasons:
1. Enhanced Detection Rates
Machine learning models can analyze vast amounts of data in real-time, allowing them to detect threats that traditional systems might miss. By recognizing behavioral patterns associated with malware, these systems can identify and neutralize threats faster and more accurately.
2. Proactive Threat Mitigation
Rather than merely reacting to known threats, machine learning empowers security systems to predict and respond to potential future attacks. This proactive approach helps businesses stay one step ahead of cybercriminals.
3. Reduction of False Positives
Traditional antivirus software often struggles with false positives, which can lead to unnecessary disruptions. Machine learning models continuously learn from new data, improving their ability to differentiate between legitimate software and malware, thus reducing the incidence of false alerts.
4. Scalability
The dynamic nature of cyber threats necessitates solutions that can easily scale. Machine learning systems can adapt to growing networks and increasing data volumes, making them suitable for businesses of all sizes.
Applications of Anti Malware Machine Learning in IT Services
As businesses increasingly migrate towards digital environments, the applications of anti malware machine learning within IT services are becoming more diverse and impactful. Here are some of the key applications:
1. Security System Deployment
Modern security systems leverage machine learning algorithms to enhance their protective capabilities. These systems can analyze network traffic, monitor user behavior, and detect unusual activity in real-time, significantly bolstering an organization's security posture.
2. Network Anomaly Detection
Machine learning can be used to develop models that monitor network activity and identify anomalies. This allows IT teams to quickly address potential breaches or malware infiltrations before they escalate into more significant issues.
3. Endpoint Security Solutions
With an increasing number of devices connecting to business networks, endpoint security has become critical. Machine learning-driven antivirus solutions can provide comprehensive protection by continuously learning from device interactions to detect and mitigate threats effectively.
4. Predictive Analytics for IT Operations
Machine learning can analyze trends and historical data to predict potential IT failures or security breaches. By understanding patterns, IT teams can take preemptive action, reducing downtime and improving overall security.
Challenges in Implementing Machine Learning for Anti Malware
Despite its advantages, there are challenges that businesses must consider when implementing anti malware machine learning solutions:
1. Data Privacy Concerns
As machine learning systems require vast amounts of data to train effectively, organizations must address privacy concerns and ensure compliance with data protection regulations.
2. Complexity of Implementation
The integration of machine learning into existing IT infrastructure can be complex and may require specialized expertise. Businesses may need to invest in training or hiring skilled personnel.
3. Evolving Cyber Threat Landscape
Cybercriminals are continually developing new techniques to circumvent security measures. Consequently, machine learning models must be regularly updated and retrained to remain effective against emerging threats.
The Future of Anti Malware Machine Learning
Looking ahead, the future of anti malware machine learning is promising. Emerging technologies such as deep learning and natural language processing are likely to enhance malware detection capabilities further. Organizations must stay informed about these advancements to maintain a competitive edge in cybersecurity.
Best Practices for Implementing Anti Malware Machine Learning Solutions
While the potential of machine learning in combating malware is vast, businesses should follow best practices for effective implementation. Here are some essential steps:
1. Assess Your Current Security Infrastructure
Before deploying machine learning solutions, conduct a thorough assessment of your existing security measures. Understanding your vulnerabilities and strengths will guide the implementation process.
2. Choose the Right Machine Learning Model
Not all machine learning models are created equal. Consider your organization’s specific needs, including volume of data, network configurations, and the nature of potential threats. Selecting the appropriate model is crucial for success.
3. Continuous Learning and Adaptation
Establish processes for ongoing learning and adaptation within your security systems. Regular updates and retraining of machine learning models are essential to counteract evolving threats.
4. Train Your Team
Your employees are your first line of defense against cyber threats. Providing training on the latest cybersecurity protocols and the role of machine learning in detecting malware can enhance your organization's overall security posture.
5. Collaborate with Experts
If your organization lacks the necessary expertise, consider engaging with cybersecurity specialists who have experience in implementing machine learning for anti malware solutions.
Conclusion
The intersection of IT services, computer repair, and security systems with anti malware machine learning holds immense potential for businesses looking to bolster their cybersecurity efforts. By understanding the intricacies of this technology, recognizing its benefits, and implementing best practices, organizations can protect their digital assets against an ever-evolving threat landscape.
As cyber threats continue to escalate, investing in advanced solutions like machine learning-powered anti malware systems will not just be an option; it will become a necessity. The future of IT is bright, and embracing machine learning is a critical step towards achieving unparalleled cybersecurity.